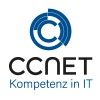
CCNet
Jul 31, 2024 • 2 min read
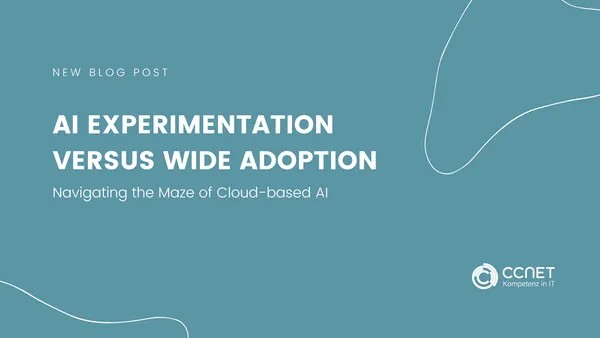
AI Experimentation versus Wide Adoption: Navigating the Maze of Cloud-based AI
Within cloud technology, artificial intelligence (AI) has taken center stage. Companies of all sizes are experimenting with AI services to push the boundaries of what is possible. However, while some organizations are still in the infancy of AI experimentation, navigating the maze of challenges, others have already taken the leap towards wide adoption. This blog sheds light on the differences between these phases, discusses the challenges of scaling AI applications versus the challenges faced during initial experimentation, and provides insights into how companies can successfully navigate the transition.
The Experimentation Phase: A Launchpad for Innovation
In the experimentation phase, companies venture into testing AI technologies, often with limited scope and specific use cases. This phase is characterized by a high willingness to experiment and learn, with the aim of exploring the potential of AI for their own business models. Cloud-based AI services such as Azure OpenAI, Google Cloud AI, and AWS SageMaker play a crucial role by simplifying access to advanced AI tools and enabling flexible scaling.
During this time, collaboration between data scientists, developers, and business strategists is crucial to drive innovation while keeping costs and resources in check. The challenge lies in finding a balance between the pursuit of new opportunities and risk mitigation.
From Experimentation to Wide Adoption
The transition from experimentation to wide adoption of AI in the cloud marks a turning point for companies. This step requires strategic planning, infrastructure scaling, and adjustment of corporate culture to make AI usage an integral part of business operations.
A key factor influencing this transition is the proof of the value of AI applications. Companies must clearly define how AI contributes to value creation, whether through efficiency improvements, generating new revenue streams, or enhancing customer interaction. This requires close collaboration between teams to develop AI models that solve real business problems and deliver measurable ROI.
Challenges in Scaling AI
Scaling AI applications poses a number of challenges, including:
- Data Security: Ensuring the security of sensitive data when using AI services is crucial.
- Complexity Management: As AI usage increases, so does the complexity of managing these systems.
- Talent Shortage: Qualified professionals are required to lead and maintain AI projects.
- Cost Control: The costs of developing and operating AI models can escalate quickly.
Success Factors for Wide Adoption
To address these challenges and successfully implement AI on a large scale, companies should consider the following steps:
- Strengthening AI Governance: Establish clear guidelines and standards for AI deployment.
- Building Expertise: Invest in training your employees and recruiting AI experts.
- Promoting an AI-friendly Culture: Create an environment that supports innovation and encourages experimenting with AI technologies.
- Implementing AI Ethics Guidelines: Ensure that your AI applications adhere to ethical principles.
Conclusion
The journey from AI experimentation to wide adoption is complex and requires careful planning and execution. Companies that successfully navigate this transition can reap the tremendous benefits of AI, from increased efficiency to revolutionary new business models. The future belongs to those who are willing to tackle the challenges of scaling AI and fully capitalize on the opportunities it offers.