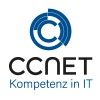
CCNet
Jul 10, 2024 • 2 min read
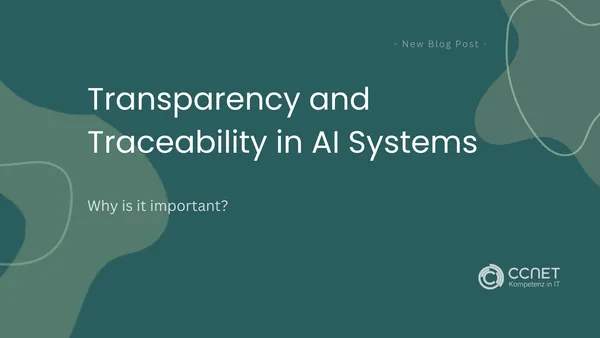
Transparency and Traceability in AI Systems: Why Is It Important?
In an increasingly digitalized world where artificial intelligence (AI) plays a growing role in decision-making, transparency and traceability in these systems are critical aspects. This is especially true in legal applications, where decisions can have far-reaching consequences, and trust in these technologies is fundamental to their acceptance. Therefore, it is essential to develop guidelines and standards to ensure comprehensive disclosure of how AI systems work and their data basis, to ensure proper auditability and accountability.
Why Is Transparency in AI Systems Important?
Transparency in AI systems means that both the workings of the systems and the decisions they make are understandable and comprehensible to users. This transparency is particularly crucial because it helps strengthen users' trust in the technology and allows for an informed review of the decisions by human users, enabling potential risks and biases to be identified and corrected in time to ensure fair and ethically responsible use.
Transparency in Decision-Making
For AI systems, ensuring transparency is often more complex because their decision logic is based on complicated algorithms that are difficult to understand. Therefore, innovative approaches such as algorithmic explainability and interpretability are crucial to strengthen users' trust and uncover potential risks.
In contrast, machine-learning systems, often described as "black boxes," pose a greater challenge. These systems can efficiently recognize complex data patterns and make decisions, but the exact mechanisms behind their decision-making are often not transparent and thus difficult to trace, complicating trust-building and ensuring accountability.
The Importance of Explainability
The explainability of AI systems is closely tied to transparency and refers to how well the system's actions can be communicated in a way that is understandable to humans. This is especially crucial in legal applications, where decisions often need to be justified, and the underlying reasoning must be disclosed to strengthen citizens' trust in the legal system and ensure a fair judiciary.
Challenges and Solutions
Creating transparency and traceability in AI systems is not without challenges. Concerns about data protection, intellectual property protection, and the technical complexity of the systems can limit the disclosure of information. Solutions could include developing standards for AI transparency, promoting open discourse about AI decisions, and involving independent reviews. Additionally, it's essential to implement mechanisms to ensure the security of sensitive data and increase transparency about the use of AI algorithms by organizations.
Conclusion
The continuous development of standards and best practices can help further improve the transparency and traceability of AI systems. This requires a joint commitment from regulators, researchers, developers, and civil society. Moreover, establishing mechanisms for independent review and audit of AI algorithms can help identify and correct potential biases or discrimination early on. Only through this holistic approach can we build trust in AI systems and ensure they are fair, reliable, and acceptable, especially in sensitive applications like legal practice.
Would you like to know more about how AI systems are developed to achieve higher transparency, or are you interested in specific technologies that can help achieve this?